How can Artificial Intelligence Help Improve Climate Forecasting and Risk Information?
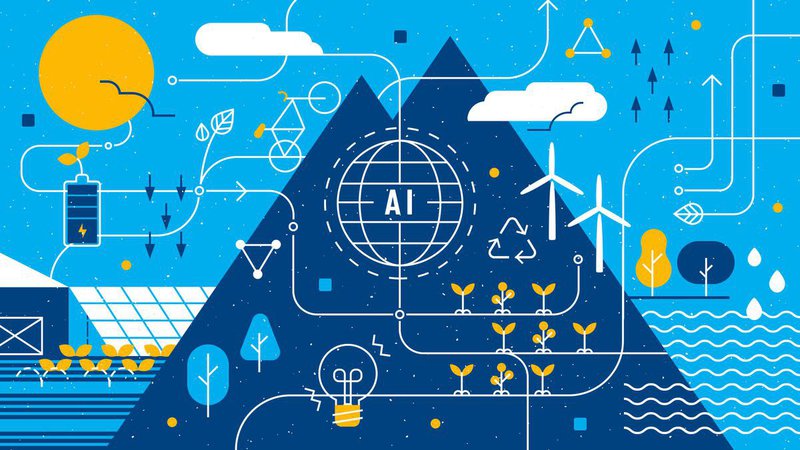
By author, Herbert Misiani, ICPAC Climate Researcher
Contributions by ICPAC Climate Change Technical Working Group
Climate is chaotic in nature, with many processes and feedbacks which have not been fully understood. Predicting the future states of such a system is often a big challenge and often requires quality long-term climate records spanning several decades. The rising global temperatures have not made things any better for climate scientists since they have already impacted different aspects of climate variability. Every day different centers around the world churn out additional petabytes worth of data; for example, data from conventional observing stations, climate simulations, satellites, and radars. The large amount of data requires novel approaches to identify hidden patterns not discoverable with conventional methods and which can help with predicting future states of the climate. One approach that is slowly gaining momentum among climate scientists is the application of Artificial Intelligence (AI).
Artificial Intelligence (AI) is a broad term that loosely describes the process of mimicking the human mind by machines to effectively learn and solve real-world problems[1]. AI is the main body where all other subsets, such as Machine Learning (ML) and Deep Learning (DL) fall. The usefulness of AI methods in climate science can be found in applications where non-conventional statistical modeling approaches used to identify atmospheric processes are limiting (for example, approaches that assume linear relationships) and in applications where analysis is computationally intensive such as parameterizing schemes which are usually embedded in dynamical models.
In fact, AI could facilitate many climate change strategies from policy and engineering, according to Climate Change AI (CCAI).
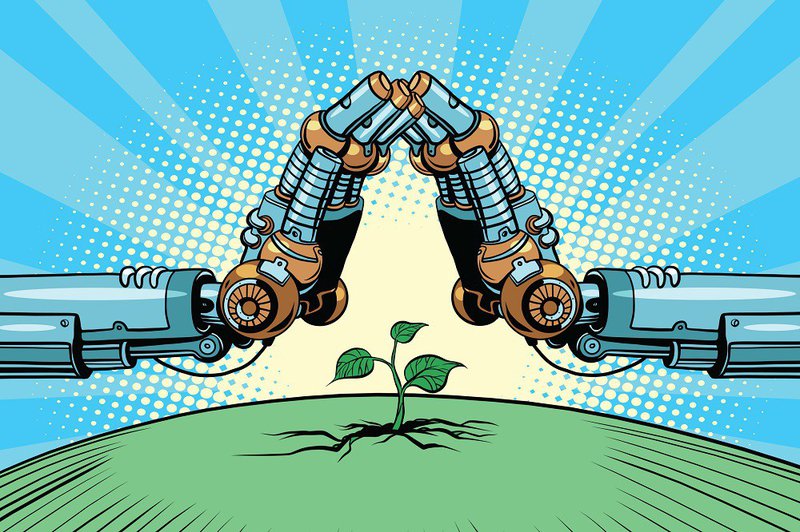
Which are some of the areas where AI is being applied in climate science?
There are quite a number of instances where the use of AI has proven useful in climate science. An example is the detection of extreme weather events (e.g.
tropical cyclones, atmospheric rivers, and weather fronts) in climate datasets using Deep Convolution Neural Network (DCNN) as well as prediction of extreme climate events[2,3]. Machine learning has also been used for attribution studies such as the attribution and prediction of seasonal precipitation and temperature trends in southeast Australia[4]. They have also been used to improve real-time decision making for high-impact weather such as storm duration, severe hail, and aviation turbulence [5,6,7].
Additionally, AI has been extensively used in climate modeling, especially in improving various aspects of the Earth System Models (ESM) such as, improving convection parameterization and their speeds of computation, and improving land-cover feedbacks through the classification of land cover from different datasets [8,9,10]. Finally, AI has been applied in improving climate datasets from different sources such as satellite rainfall estimates (e.g. PERSIANN), improving estimates of min/max temperature data for incomplete time series, and downscaling climate models for impact assessment [11,12,13]. However, beyond climate forecasting, AI is also being used to improve impact-based forecasting, establishing thresholds for alerts, or integrating customer feedback to improve climate service.
Challenges
As demonstrated, there are quite a number of areas within climate science where AI has been successfully applied. However, some scientists still have reservations when it comes to AI. One of the reservations is that the models are black boxes and it is difficult to understand what types of decisions are made by the machine and why. The other challenge is that some algorithms are still computationally intensive such as Deep Learning (DL) and require a lot of data for training and validating the models. For the Eastern African region where observation records are sparse and cover short time-periods, understanding the observed patterns and interactions between variables, therefore becomes quite a challenge with this limited dataset.
Opportunities
Given that AI algorithms are relatively less computationally intensive compared to dynamical approaches, they can be used to provide early warning information on high impact weather and climate events in institutions where computational resources are limited. Further still, there are even more areas in climate science and other fields where AI can be applied to bring out new insights that would otherwise be a challenge to achieve through conventional methods. One interesting area is the application of AI tackling climate change by improving how climate information is generated and disseminated. AI could also play a role in monitoring the impact of climate variability and change by observing real-time food shortage risks or changes in the ecosystem through the use of remote sensing data.
The other interesting area is in agricultural applications where AI has been used to, among other things, automate irrigation systems; connecting farmers to credit services, and provide farmers with a decision support tool which helps them with critical decisions such as when to plant; deciding on drought-tolerant crops, and crop suitable for particular regions. For example, Apollo Agriculture which is a company based in Kenya uses machine learning and satellite data to provide farmers with better credit decisions and automate farm processes with the goal of minimizing costs. Farm drive, which is also another company based in Kenya is using machine learning methods to unlock access to credit for smallholder farmers.
The use of AI in disaster response is emerging. In fact sites like Artificial Intelligence for Disaster Response (AIDR) analyze large volumes of social media data in real-time during humanitarian crises in an effort to prevent massive loss of lives while at the same time attempting to make rescue efforts easy and efficient. During a flood, for example, AI algorithms assess the extent of the flooding, identify damaged buildings and roads through satellite imagery, which could help in identifying those in danger, give information on possible escape routes, and increase the efficiency of emergency aid distribution.
Reference
- Rolnick, D., Donti, P. L., Kaack, L. H., Kochanski, K., Lacoste, A., Sankaran, K., Ross, A.S., Milojevic-Dupont, N., Jaques, N., Waldman-Brown, A. and Luccioni, A., (2019). Tackling climate change with machine learning.arXiv preprint arXiv:1906.05433.
- Liu, Y., Racah, E., Correa, J., Khosrowshahi, A., Lavers, D., Kunkel, K., Wehner, M. & Collins, W. (2016). Application of deep convolutional neural networks for detecting extreme weather in climate datasets. arXiv preprint arXiv:1605.01156.
- Deo R C and Sahin M (2015) Application of the extreme learning machine algorithm for the prediction of monthly Effective Drought Index in eastern Australia Atmos. Res. 153 512–25
- Hartigan, J., MacNamara, S., & Leslie, L. M. (2020). Application of Machine Learning to Attribution and Prediction of Seasonal Precipitation and Temperature Trends in Canberra, Australia. Climate, 8(6), 76.
- McGovern, A., Elmore, K. L., Gagne, D. J., Haupt, S. E., Karstens, C. D., Lagerquist, R., Smith, T. & Williams, J. K. (2017). Using artificial intelligence to improve real-time decision-making for high-impact weather. Bulletin of the American Meteorological Society, 98(10), 2073–2090.
- Gagne, D. J., McGovern, A., Haupt, S. E., Sobash, R. A., Williams, J. K., & Xue, M. (2017). Storm-based probabilistic hail forecasting with machine learning applied to convection-allowing ensembles. Weather and forecasting, 32(5), 1819–1840.
- Liu, Y. Y., Li, L., Liu, Y. S., Chan, P. W., & Zhang, W. H. (2020). Dynamic spatial-temporal precipitation distribution models for short-duration rainstorms in Shenzhen, China based on machine learning. Atmospheric Research, 237, 104861.
- Gentine P, Pritchard M, Rasp S, Reinaudi G and Yacalis G 2018 Could machine learning break the convection parameterization Deadlock? Geophys. Res. Lett. 45 5742–51
- Krasnopolsky V M, Fox-Rabinovitz M S and Chalikov D V (2005) New approach to calculation of atmospheric model physics: Accurate and fast neural network emulation of longwave radiation in a climate model Mon. Weather Rev. 133 1370–83
- Rodriguez-Galiano V F, Ghimire B, Rogan J, Chica-Olmo M and Rigol-Sanchez J P (2012) An assessment of the effectiveness of a random forest classifier for land-cover classification ISPRS J. Photogramm. Remote Sens. 67 93–104
- Hsu K L, Gao X G, Sorooshian S and Gupta H V (1997) Precipitation estimation from remotely sensed information using artificial neural networksJ. Appl. Meteorol. 36 1176–90
- Richard M, McKinnon K A and Pillai N 2018 Bias correction in daily maximum and minimum temperature measurements through Gaussian process modeling arXiv:1805.10214v2
- Salvi K, Villarini G and Vecchi G A 2017 High resolution decadal precipitation predictions over the continental United States for impacts assessment J. Hydrol. 553 559–73
- Hong Y, Gochis D, Cheng J-T, Hsu K-L and Sorooshian S (2007) Evaluation of PERSIANN-CCS rainfall measurement using the NAME event rain Gauge Network J. Hydrometeorol. 8 469–82