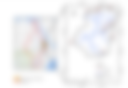
AD-vine copula-based quantile regression towards merging satellite precipitation products over rugged topography: a case study in the upper Tekeze–Atbara Basin
Precipitation is a vital key element in various studies of hydrology, flood prediction, drought monitoring, and water resource management. The main challenge in conducting studies in remote regions with rugged topography is that weather stations are usually scarce and unevenly distributed.
However, open-source satellite-based precipitation products (SPPs) with a good resolution offer other choices in these areas with little data, which usually means a lot of uncertainty.
We have come up with a D-vine copula-based quantile regression (DVQR) model to combine multiple SPPs with rain gauges (RGs) to make each satellite product less uncertain.
To study the DVQR model during the summer monsoon seasons of 2001–2017, it was compared to two other quantile regression methods based on the multivariate linear (MLQR) and the Bayesian model averaging (BMAQ) techniques. It was also compared to two traditional merging methods based on descriptive and categorical statistics: the simple modeling average (SMA) and the one outlier-removed average (OORA). Four SPPs have been considered in this study, namely, Tropical Applications of Meteorology using SATellite (TAMSAT v3.1), the Climate Prediction Center MORPHing Product Climate Data Record (CMORPHCDR), Global Precipitation Measurement (GPM) Integrated Multi-satellite Retrievals for GPM (IMERG v06), and Precipitation Estimation from Remotely Sensed Information using Artificial Neural Networks (PERSIANN-CDR). The bilinear (BIL) interpolation technique was applied to downscale SPPs from a coarse to a fine spatial resolution (1km).
The rugged topography region of the upper Tekeze-Atbara Basin (UTAB) in Ethiopia was selected as the study area.
The results show that the DVQR, MLQR, BMAQ, and traditional merging methods do a better job of estimating precipitation data than the downscaled SPPs.